All published articles of this journal are available on ScienceDirect.
A Bioinformatics and Systems Biology Approach to Identifying the Influence of COVID-19 on Patients with Osteoarthritis
Abstract
Background
Clinical findings show that the incidence of osteoarthritis (OA) is significantly increased in the late stage of coronavirus disease 2019 in southwest China. However, there continues to be a lack of substantial evidence elucidating the potential molecular mechanism underlying OA and COVID-19.
Objective
The objective of this study is to clarify the genetic interaction between OA and COVID-19 and to explore the therapeutic targets for COVID-19-infected patients with OA.
Methods
Two differentially expressed gene (DEG) sets extracted from the GSE171110 and GSE55235 datasets were intersected to generate common DEGs, which were used for functional enrichment, hub gene and pathway identification, and candidate drug-gene and disease–gene prediction. Associations between hub genes and immune cell infiltration were also generated to increase the clinical relevance.
Results
A total of 127 DEGs were screened from the COVID-19 and OA datasets. Functional enrichment analysis demonstrated that the common pathways activated in both diseases were mainly concentrated in the circadian rhythm, cellular response to chemical stimulus, B cell signaling pathway, and response to organic substances. The TF and miRNA analyses identified 14 TFs and 2 genes (CDK1 and TOP2A) as final hub genes. Furthermore, 28 possible drugs targeting these 2 hub genes and 9 relevant tumor diseases were predicted for the treatment of COVID-19-infected patients with OA. CDK1 and TOP2A were successfully identified as hub genes that can serve as novel therapeutic targets for patients with COVID-19. We also screened out 28 potential drugs and 9 diseases valuable for the treatment of OA after COVID-19 infection.
Conclusion
CDK1 and TOP2A were successfully identified as hub genes that can serve as markers of novel targeted therapy for patients with OA after COVID-19 infection. Additionally, 1 immune cell (resting mast cells), 9 tumor diseases, and 28 potential drugs were identified for the potential therapeutic treatment of OA after COVID-19 infection.
1. INTRODUCTION
Osteoarthritis (OA) is a degenerative pathology with progressive inflammation and can result in cartilage loss, synovial inflammation, subchondral bone remodeling, and knee dysfunction, seriously affecting the health and quality of life of patients [1]. The global number of patients suffering from this disease exceeds 500 million, which equates to 7% of the world’s population [2].
The progression of OA is a complicated pathological process that is associated with multiple risk factors, such as trauma, aging, obesity, infection, and metabolic disease [3]. In recent years, mounting evidence confirms that the regulations of specific genes are involved in the progression of OA through diverse genetic analyses and boost the development of multiple drugs targeting these genes for the treatment of OA. For example, a recent study validated FoxO1’s defense against oxidative stress in osteocytes, and that applying HDAC inhibitors effectively alleviates osteoarthritis via targeting FoxO transcription factors [4]. Nevertheless, the specific mechanism underlying OA remains undefined. Therefore, it is of great interest to delve into the molecular mechanism with which OA complies.
Coronavirus disease 19 (COVID-19) is an infectious disease caused by the SARS-CoV-2 virus. Coronaviruses are a family of positive-sense, enveloped RNA viruses that cause various illnesses in mammals and birds. The human coronaviruses typically cause mild upper respiratory infection and are responsible for approximately 15%–30% of all cases of the common cold in adults and children. Early symptoms usually present with fatigue, fever, dry cough, and anosmia. Approximately 80% of cases are mild and self-limited, primarily affecting the upper airway with limited involvement of the lungs. However, severe infection, characterized by dyspnea, tachypnea, hypoxemia, cardiovascular sequalae, and extensive lung disease, occurs in about 15% of cases [5]. Therefore, further research into the biology, epidemiology, and medical management of COVID-19 is crucial to prevent the global outbreak of these severe diseases.
An interesting phenomenon was found during the second wave of the COVID-19 pandemic (Omicron BA. 5.2) in China from October to December 2022. Musculoskeletal symptoms appeared to be some of the major discomforts, including musculoskeletal pain, arthralgia, and inflammation in synovial joints, in addition to the classical symptoms of the virus, such as acute fever, headaches, a cough, dyspnea, and pneumonia [6]. In particular, older adults infected with COVID-19 commonly suffer from more severe joint pain than others [7]. However, the connection between COVID-19 and arthralgia caused by osteoarthritis (OA) remains unclear. Therefore, it is necessary to reveal the intrinsic molecular mechanism between COVID-19 and OA.
Previous studies have shown that patients with COVID-19 are often accompanied by a high level of pro-inflammatory cytokines, such as interleukin-1, which evolve into a cytokine storm [8]. Furthermore, an abnormally high level of ROS accumulation resulting from elevated inflammatory cytokines, including IL-1β, IL-6, and TNF-α, was validated to promote oxidative damage, which induces the death of synovium cells and finally exacerbates OA [9]. However, the common pathologic factors of OA and COVID-19 are yet to be elucidated. Therefore, it is necessary to investigate the common pathways in both diseases and potential small-molecule chemical compounds for the treatment of OA patients with COVID-19.
In this study, we used gene expression profiling and microarrays from different data reservoirs to identify 127 common DEGs of OA and COVID-19 and investigated the potential common pathways activated in both diseases through multiple bioinformatics analyses, including pathway and GO functional enrichment analyses. Furthermore, we constructed a PPI network to screen out the possible hub genes that can act as therapeutic targets. Afterward, analyses of transcriptional factors (TFs) and miRNAs were performed based on the common DEGs. Finally, through drug-gene and disease–gene interaction analyses, we predicted drug-targeting common hub genes to explore the potentially available small molecular compounds for the treatment of COVID-19-infected OA patients. The organizational diagram of this study has been presented as a graphical abstract.
2. MATERIALS AND METHODS
2.1. Dataset Collection
To explore the association between osteoarthritis and COVID-19, we downloaded corresponding microarrays and RNA-seq datasets from the NCBI GEO database (Gene Expression Omnibus, GEO, http://www.ncbi .nlm.nih.gov /geo/). The osteoarthritis dataset (GSE55235, Homo sapiens, https://www.ncbi .nlm.nih. gov/geo/query /acc.cgi?acc= GSM1332220) comprised 10 osteoarthritis samples, 10 normal controls, and GPL96 [HG-U133A] Affymetrix Human Genome U133A Array. The COVID-19 dataset (GSE171110, Homo sapiens, https://www.ncbi .nlm.nih.gov /geo/query/acc. cgi?acc= GSE171110) comprised 44 COVID-19 samples, 10 healthy controls, and GPL16791 Illumina HiSeq 2500. Then, we converted the probes into gene symbols one by one by using the corresponding mRNA probe expression matrix and annotation files of the two datasets obtained from the sequencing platform. After removing the probes without a gene symbol to match, we generated a gene expression matrix for the subsequent analyses. Regarding the different probes that mapped the same gene, we calculated the mean value of these probes as the expression value of this gene.
2.2. Screening of Disease-Associated Differentially Expressed Genes
To screen the disease-associated differentially expressed genes (DEGs), we performed DEG analysis for the normal and diseased samples from both the GSE55235 and GSE171110 datasets. Hierarchical clustering was applied in this study to assess the expression of different genes in the COVID-19 (GSE171110) and OA (GSE55235) datasets. The corresponding p-value and logFC of each gene were calculated using the “limma” package of R software (http:// bioconductor.org /packages/release /bioc/html/ limma.html, version 3.48.3) and corrected by the BH method. We evaluated the expression differences by the fold change and significance and set the differential expression cutoff as follows: adjP.Value < 0.05 and |logFC| > 0.263 (i.e., 1.2-fold change).
2.3. Identification of Common DEGs among OA and COVID-19
Based on the DEGs screened out from the previous step, we generated Venn diagrams using the “VennDetail” package in R software (version 1.2.0,https://github.com /guokai8/ VennDetail) to identify the common DEGs of osteoarthritis and COVID-19 for subsequent analyses.
2.4. Functional Enrichment Analysis of Common DEGs
Functional enrichment analysis was performed based on the common DEGs from the previous step. We capitalized on the online gprofiler tool (https://biit. cs.ut.ee /gprofiler/ convert) to investigate the functions of these common DEGs and conducted pathway and Gene Ontology (GO) function enrichment analyses using over-representation analysis (ORA) in the functional profiling module of gprofiler. The GO functional enrichment analysis incorporated three categories: Biological process (BP), cellular component (CC), and molecular function (MF). Meanwhile, the pathway enrichment analysis incorporated three patterns: wikipathway, reactome pathway, and KEGG human pathway. The cutoff was p < 0.05, adjusted by the BH method.
2.5. Protein-Protein Interaction Network Analysis
We constructed a protein-protein interaction (PPI) network of common DEGs through the STRING database (Version: 11.5, http://string-db.org/) with an interaction score set to 0.7. CytoHubba is a powerful plugin that contributes to finding the key elements in a complicated network. We adopted four topological algorithms from the cytoHubba plugin, namely, MCC, MNC, Degree, and EPC, to predict and reveal the top 15 important hub genes. The intersection of the outcomes generated by the four algorithms was defined as candidate hub genes.
2.6. Association between Hub Genes and Immune Cell Infiltration
Through CIBERSORT, we computed the number of immune cells in both disease datasets to reveal the association between the hub genes and immune infiltration. CIBERSORT is an algorithm that can quantify the abundance of specific cell types. The flow cytometry sorting experiment successfully validated and evaluated the composition of the immune cells in the samples and generated the proportions of 22 types of immune cells in each sample. We further analyzed the correlations between candidate hub genes and 22 types of immune cells using Spearman’s correlation and selected the immune cells that correlated with both diseases to depict a correlation scatterplot.
2.7. Prediction of the Upstream miRNAs of Key Genes
We input the candidate hub genes into the gene module of the miRWalk 3.0 website (http://zmf.umm. uni-heidelberg. de/apps/zmf/ mirwalk3. html) and selected human species to obtain a list predicting the miRNA–target regulatory relation pairs. Based on this list, we identified the regulatory relation pairs that also existed in the TargetScan (http://www. targetscan. org/) and miRTarBase (http:// mirtarbase. cuhk.edu. cn/php/ index.php) databases and screened out the mRNA–miRNA regulatory pairs with a score of >0.95 to construct an miRNA regulatory network.
2.8. Prediction Analysis of Transcriptional Factors
We conducted a prediction analysis of the transcriptional factors for the candidate hub genes through the online database TRRUST (https://www. grnpedia.org /trrust/) to predict the TF–mRNA relation pairs and potential modes of action, such as activation, repression, or unknown. The TRRUST database ensured that all TF–target regulatory relation pairs were verified by the experiments through MeSH term searching and an improved sentence-based text-mining algorithm, as well as through manual proofreading after mining. Then, with these predicted results, we constructed a TF–target network.
2.9. Gene Set Enrichment Analysis of Hub Genes
We defined the genes that existed in the TF–target network or miRNA regulatory network as final hub genes and investigated the differentially regulatory pathways between the high- and low-expression groups of final hub genes through the gene set enrichment analysis (GSEA) algorithm before identifying the signaling pathways activated in both diseases. The pathways that belonged to the intersection of the GSEA results of both diseases and also correlated with the high expression of the final hub genes were considered activated pathways. The cutoff for significant enrichment was set to p < 0.05.
2.10. Drug–Gene and Disease–Gene Interaction Prediction
The DGIdb database (Drug-Gene Interaction database,http://dgidb.org/) is an open resource project for researchers to browse, search, and screen drug-gene interaction information and druggable genes. The search range in the drug prediction of this study included 20 commonly used drug databases. The selection of gene type and interaction was set as “Approved.” Finally, the DGIdb database predicted all gene-related drug-gene relation pairs and constructed a network through Cytoscape.
The DisGeNET database (http://www. disgenet.org /web/DisGeNET /menu/home ) includes and integrates genes and mutation sites associated with human disease from multiple platforms. We extracted the hub gene-disease pairs with a gda score >0.3 from this database to construct a disease–gene network.
3. RESULTS
3.1. Screening of Disease-Associated Differentially Expressed Genes
We performed DEG analysis based on the gene expression matrixes of the GSE55235 and GSE171110 databases. The cutoff for screening DEGs was set as follows: adjP.Value < 0.05 and |logFC| > 0.236 (i.e., 1.2 fold change). Fig. (1A and B) show the hierarchical clustering analyses based on the OA and COVID-19 datasets. The volcano plots, as shown in Fig. (1C and D), represent the DEGs between the normal and diseased samples from the GSE55235 and GSE171110 datasets. The number of upregulated and downregulated genes from the GSE55235 dataset was 944 and 629, respectively. Regarding the GSE171110 dataset, the number of upregulated and downregulated genes was 3653 and 4605, respectively.
3.2. Identification of Common DEGs for the Two Diseases
We generated Venn diagrams based on the DEGs screened out from the previous step. Fig. (1E) suggests 4478 and 502 downregulated DEGs from the GSE171110 and GSE55235 datasets, respectively. There were 127 common downregulated DEGs identified from the intersection of these two diagrams. Likewise, Fig. (1F) suggests 3449 and 740 upregulated DEGs from the GSE171110 and GSE55235 datasets, respectively. There were 204 common upregulated DEGs identified from the intersection of these two diagrams.
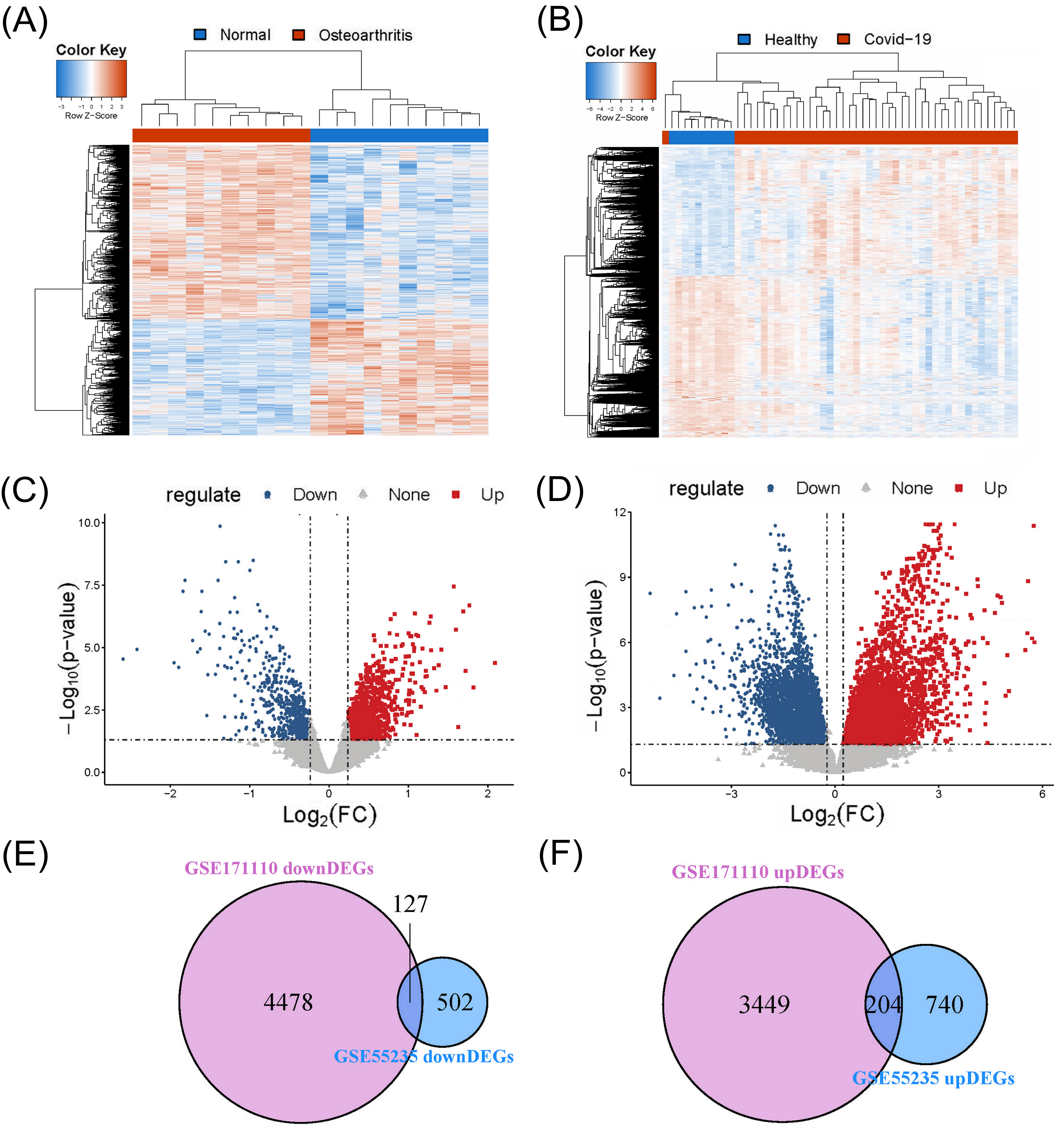
3.3. Functional Enrichment Analysis of Common DEGs
We performed functional enrichment analyses based on the downregulated and upregulated common DEGs obtained from the previous step, respectively. Through gprofiler, we used pathway and GO function enrichment analyses to investigate the biological relationships between the common DEGs and pathways. According to the p-value rank, we screened out the top five pathways from the results of each analysis.
As shown in Fig. (2A and B), KEGG human enrichment analysis of the common downregulated DEGs suggested that the top three pathways were the circadian rhythm, spliceosome, and TGF-beta signaling pathway. For the reactome pathway analysis, the top three pathways were the Transport of Mature Transcript to Cytoplasm, the Transport of Mature mRNA derived from an Intron−Containing Transcript, and mRNA 3'−end processing. Through wikipathway analysis, we found only one pathway, the TGF-beta signaling pathway, which was significantly correlated with the common downregulated DEGs. Meanwhile, the GO functional enrichment analysis of the common downregulated DEGs (Fig. 2C, D) suggested that the cellular response to chemical stimulus, negative regulation of cellular processes, and regulation of multicellular organismal processes were the top three pathways in the BP category. For the CC category, the top three pathways were nucleoplasm, nuclear speck, and nuclear body. For the MF category, the top three pathways were blue light photoreceptor activity, organic cyclic compound binding, and activin binding.
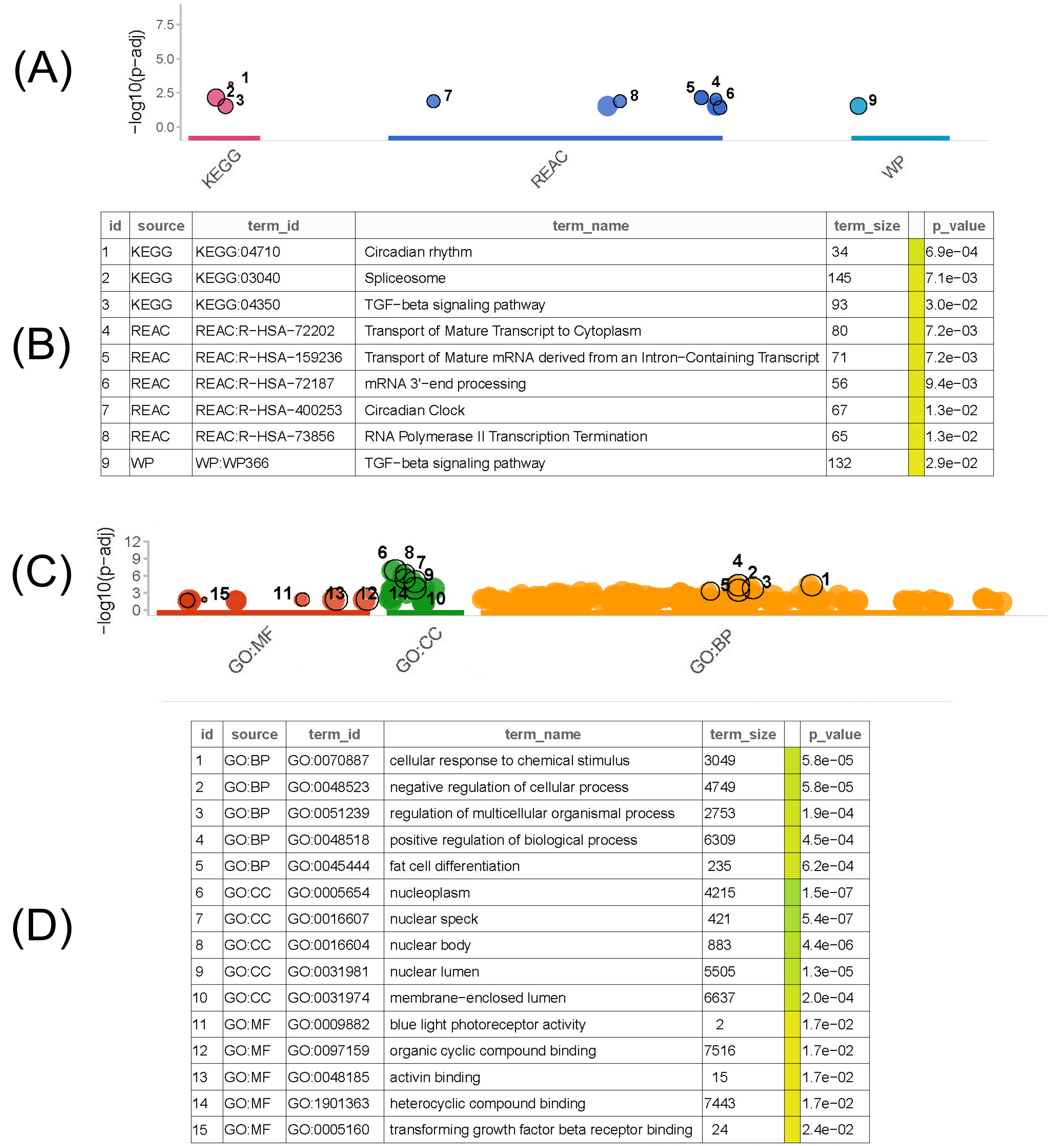
As shown in Figs. (3A and B), KEGG human enrichment analysis of the common upregulated DEGs suggested that the top three pathways were the B cell receptor signaling pathway, proteoglycans in cancer, and lysosomes. For the reactome pathway analysis, the top three pathways were neutrophil degranulation, immune system, and innate immune system. For the wikipathway analysis, the top three pathways were burn wound healing, microglia pathogen phagocytosis pathway, and TYROBP causal network in microglia. Meanwhile, the GO functional enrichment analysis of the common upregulated DEGs (Fig. 3C, D) suggested that the response to organic substances, response to stimuli, and inflammatory response were the top three pathways in the BP category. For the CC category, the top three pathways were vesicles, extracellular regions, and extracellular organelles. For the MF category, the top three pathways were protein binding, identical protein binding, and protein-containing complex binding.
3.4. PPI Network Construction of Common DEGs and Identification of Candidate Hub Genes
We constructed a PPI network of common DEGs through the STRING database and adopted four topological algorithms from the cytoHubba plugin, namely, MCC, MNC, Degree, and EPC, to reveal the top 30 key genes in the PPI network (Fig. 4A). As shown in Fig. (4B), three genes in the intersection of the results of the four algorithms were regarded as candidate hub genes, i.e., CDK1, TOP2A, and NUSAP1. Furthermore, these three hub genes exhibited significantly upregulated expression in both the OA and COVID-19 groups compared to the control group, as shown in Figs. 4C and D.
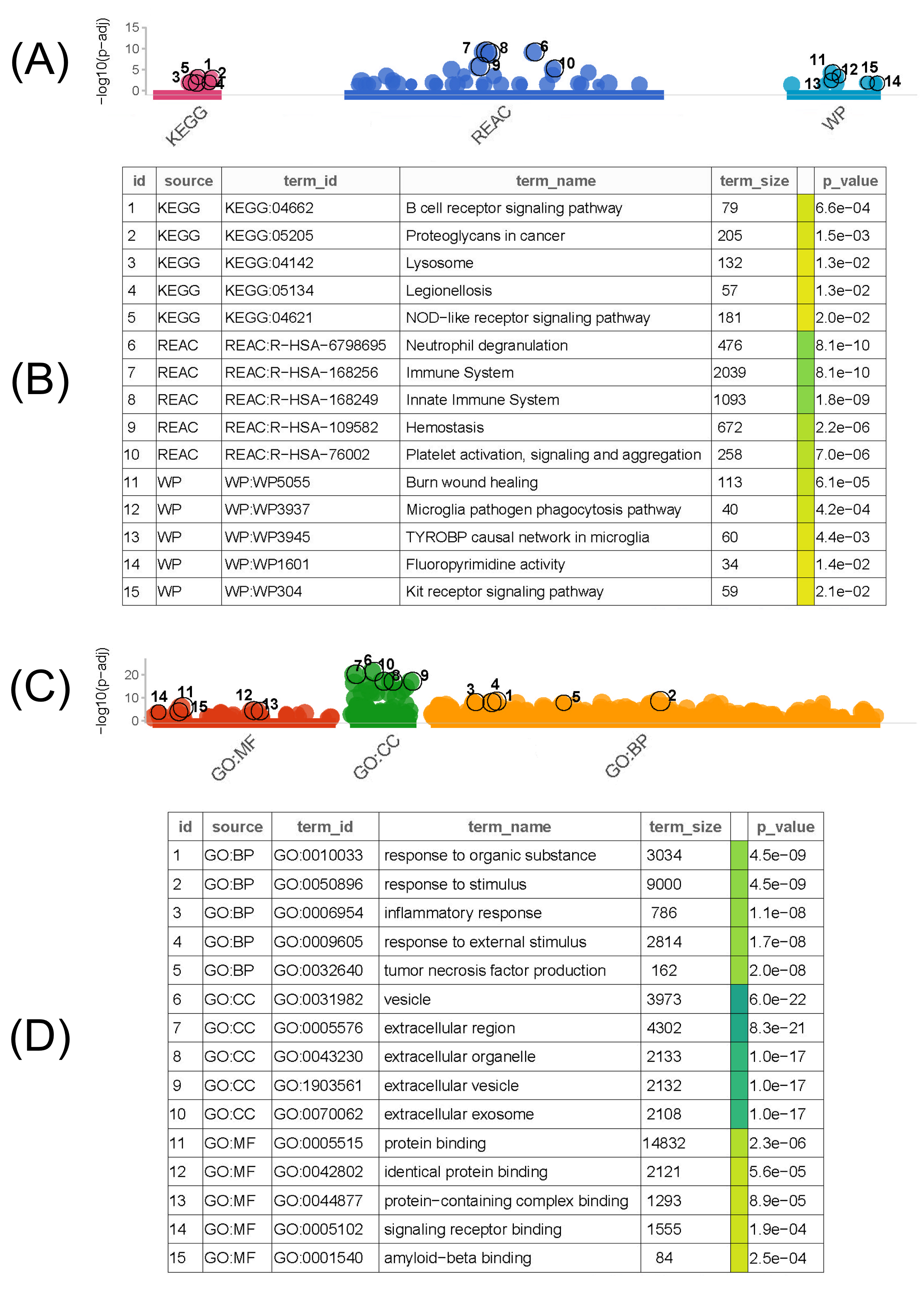
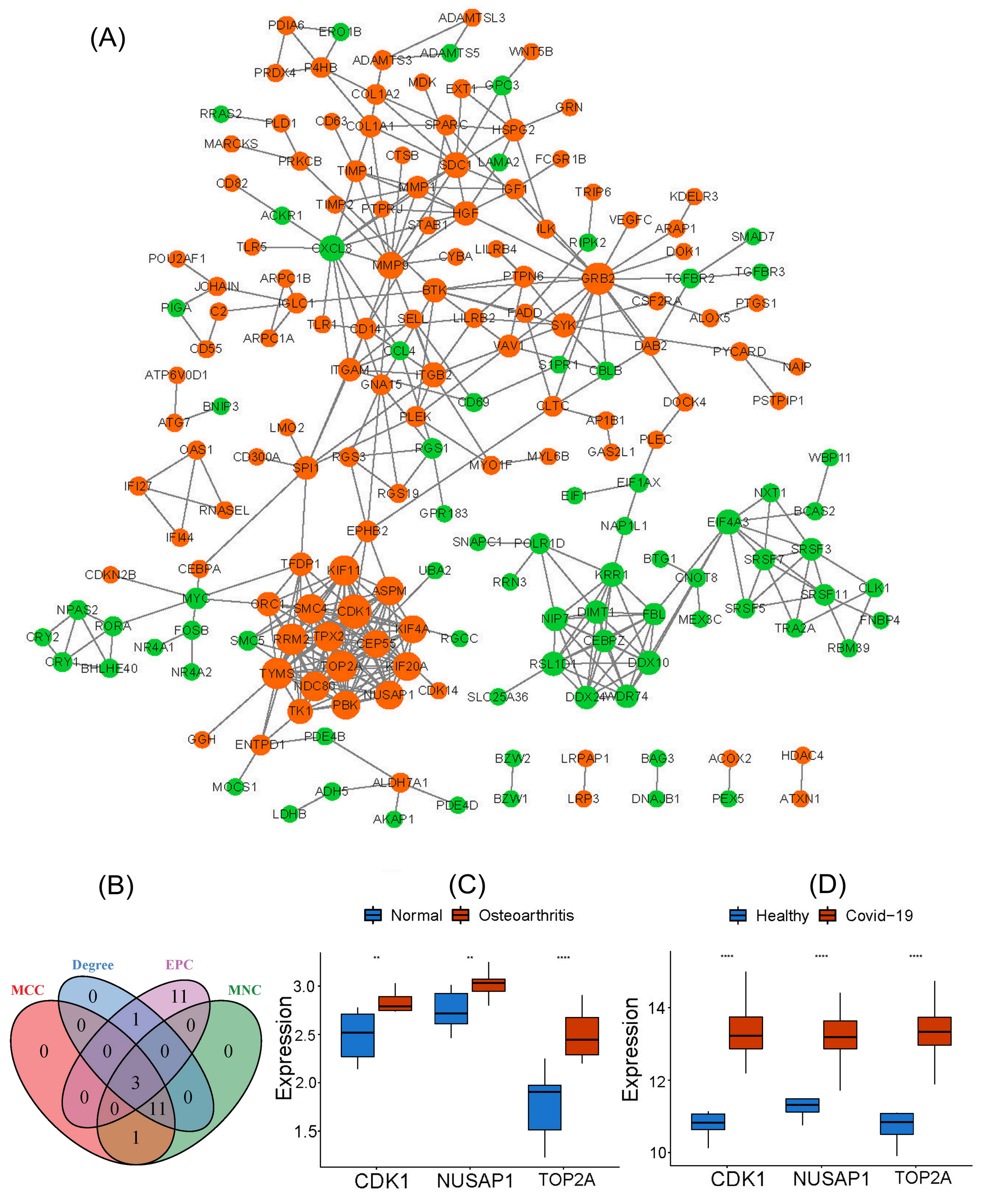
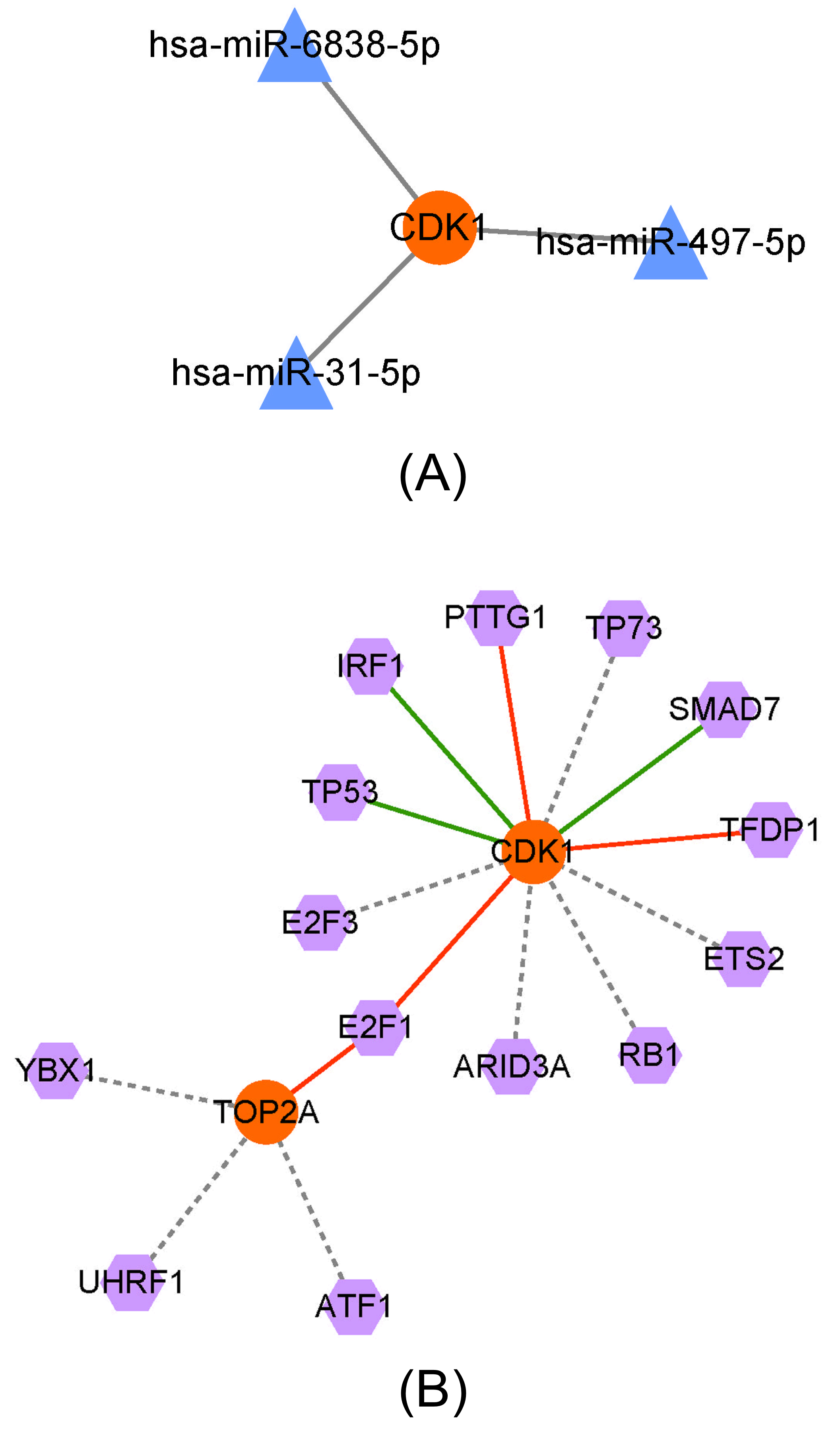
3.5. Prediction of the Upstream miRNAs of Key Genes and TF Analysis
Through the miRWalk 3.0 website, we constructed a miRNA–target network consisting of three miRNAs, one hub gene, and three regulatory relation pairs. CDK1 could interact with has-miR-6838-5p, has-miR-497-5p, and has-miR-31-5p, as shown in Fig. (5A).
We performed TF analysis of the candidate hub genes to construct a TF–target network consisting of 14 TFs, two hub genes, and 15 regulatory relation pairs. As shown in Fig. (5B), CDK1 was activated by PTTG1, TFDP1, and E2F1 but repressed by IRF1, TP53, and SMAD7. The TOP2A was activated only by E2F1. The relationships between the other TFs and these two hub genes remain unknown.
3.6. Drug–gene and Disease–gene Interaction Analyses
As described previously, we conducted drug-gene and disease–gene interaction prediction for two hub genes and constructed an interaction network consisting of two genes, 28 drugs, nine diseases, and 38 relationship pairs.
The drug-gene and disease–gene interaction analyses (Fig. 6) indicated that TOP2A was correlated with eight diseases (malignant neoplasm of the breast, breast carcinoma, liver carcinoma, malignant neoplasm of the prostate, glioma, leukemia, diffuse large B cell lymphoma, adenocarcinoma of the lung (disorder), and adrenocortical carcinoma) and could interact with 22 drugs, as follows: DIGITOXIN, DOXORUBICIN HYDROCHLORIDE, ETOPOSIDE PHOSPHATE, VINCRISTINE, IDARUBICIN, EPIRUBICIN, VALRUBICIN, TENIPOSIDE, AMSACRINE, DEXRAZOXANE, HYDROQUINONE, DAUNORUBICIN CITRATE, IDARUBICIN HYDROCHLORIDE, PACLITAXEL, FLUOROURACIL, DAUNORUBICIN HYDROCHLORIDE, ETOPOSIDE, DAUNORUBICIN, MITOXANTRONE, MITOXANTRONE HYDROCHLORIDE, DOXORUBICIN, and PODOFILOX. CDK1 was correlated with two diseases (liver carcinoma and diffuse large B cell lymphoma) and could interact with six drugs, as follows: SERTRALINE, RUCAPARIB, FENOFIBRATE, CINNARIZINE, CLOFIBRATE, and CLOTRIMAZOLE.
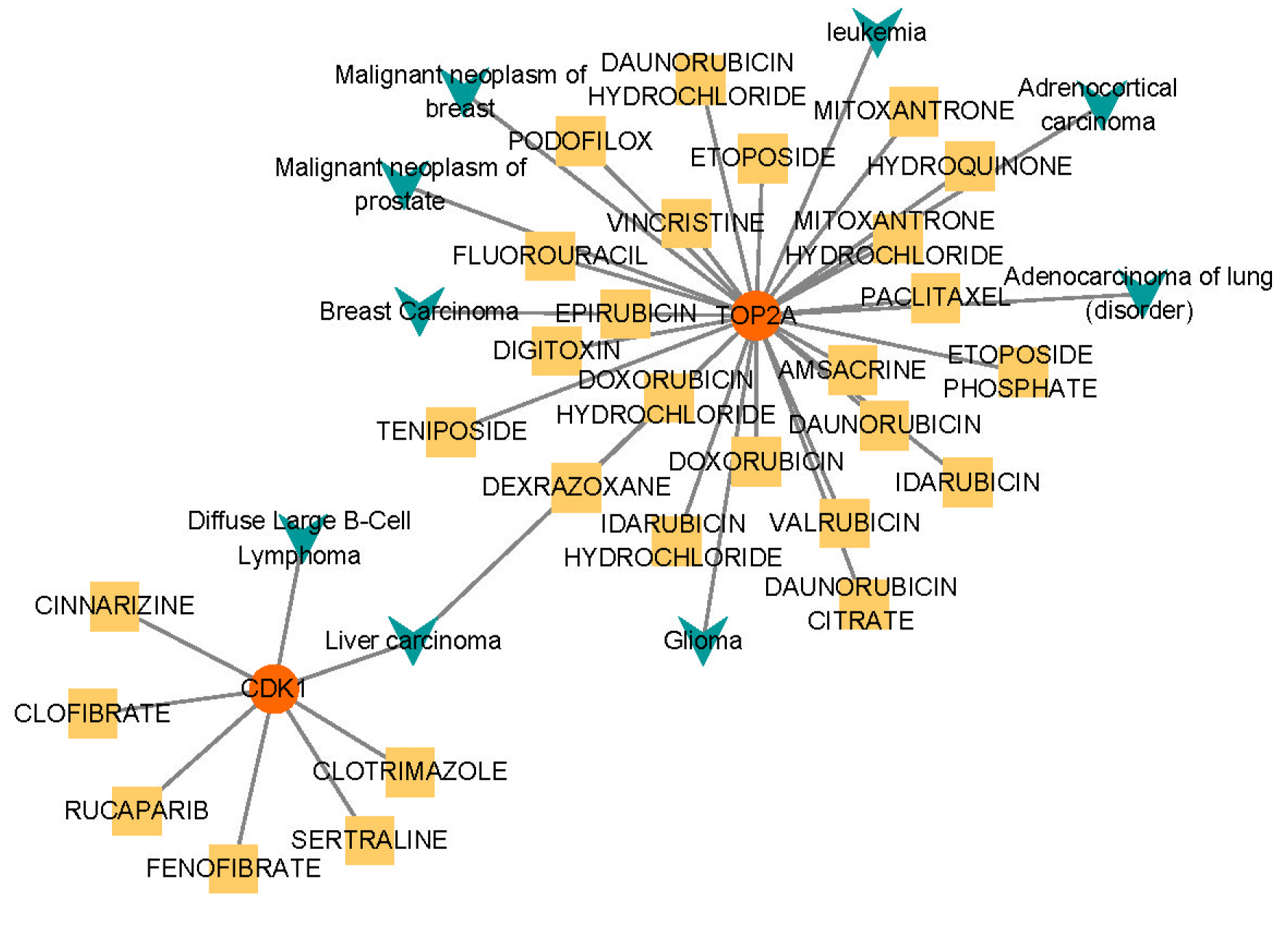
3.7. Correlations between Hub Genes and Immune Cell Infiltration
Using the CIBERSORT algorithm, we quantified the immune cells in both disease datasets and determined those immune cells with high immune infiltration by comparing the disease and control groups. Then, we investigated the correlations between 22 types of immune cells and candidate hub genes through Spearman’s correlation analysis. As shown in Figs. (7A and B, the box plots suggest that the resting mast cells in both disease groups showed a significantly higher amount of immune infiltration than in the control groups. The Spearman’s correlation analysis in Figs. (7C and D suggests that only the resting mast cells showed a significant positive correlation with three hub genes (CDK1, TOP2A, and NUSAP1) in both disease datasets (p < 0.05). The correlation scatterplots (Fig. 7E, F) show the correlations between the resting mast cells and hub genes in the OA and COVID-19 datasets.
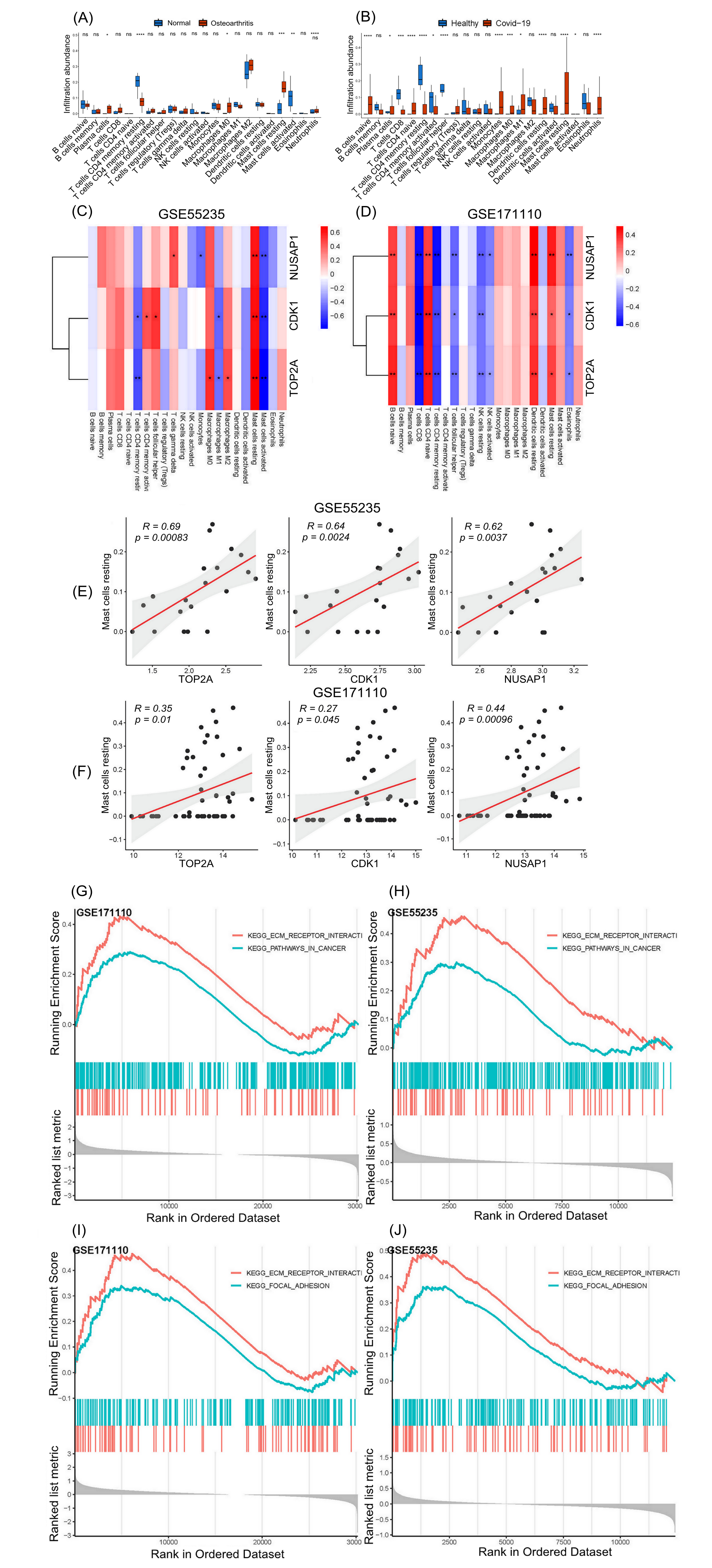
3.8. GSEA of Hub genes
We adopted the GSEA algorithm to investigate the differentially regulated pathways between the high- and low-expression groups of the final hub genes (CDK1 and TOP2A) and then identified the pathways activated in both diseases. Those pathways that were significantly correlated with a high expression of the final hub genes were considered activated pathways.
The samples were divided into two groups for GSEA according to the median value of the hub genes’ expression. As shown in Fig. (7G, H), two common pathways were enriched in osteoarthritis and COVID-19 for CDK-1: “KEGG_ECM_RECEPTOR_INTERACTION” and “KEGG_PATHWAYS_IN_CANCER” As shown in Figs. (7I and J), two common pathways were enriched in osteoarthritis and COVID-19 for TOP2A: “KEGG_ECM_RECEPTOR_INTERACTION” and “KEGG_FOCAL_ADHESION”.
4. DISCUSSION
The cross-talk between various independent diseases is attracting increasing attention and may contribute to an in-depth insight into the occurrence and progression of such diseases [10, 11]. Since the onset of the COVID-19 pandemic, global public health, food security, and individual quality of life have been in jeopardy. Another global concern is OA, a chronic musculoskeletal disease that threatens the mobility of patients, which may result in disability during aging [12, 13]. Early studies in the COVID-19 pandemic showed that joint or chest pain increased after long COVID-19 infection [14-16]. However, the specific interaction between COVID-19 and OA remains unclear.
4.1. Hypothesis and Implementation
The present study sought to reveal the pathologic associations between COVID-19 and OA in public datasets available from the NCBI GEO database. We conducted differential analysis to investigate the DEGs of the COVID-19 and OA datasets and to identify two common DEGs as hub genes, namely, CDK1 and TOP2A, by intersecting the analytical results of the two datasets.
Cell cycle kinase 1 (CDK1), also known as cyclin-dependent kinase 1, plays a crucial role in the regulation of the cell cycle and is validated to be upregulated in the peripheral blood mononuclear lymphocytes of COVID-19-infected patients. It may be associated with a heavy reduction in the immune cells of patients, exacerbating the progress of COVID-19 [17, 18][REMOVED HYPERLINK FIELD]. The CDK1 signaling pathway can promote cartilage repair and chondrocyte proliferation [19]. Topoisomerase IIa (TOP2A) is an enzyme present in the nucleus that can regulate DNA double-strand breaks and binding during cell division [20]. Furthermore, when cartilage and subchondral bone are injured, a characteristic feature of osteoarthritis, TOP2A, shows elevated upregulation in chondrocytes, which indicates a reduction in cartilage and bone regeneration [21]. Meanwhile, TOP2A has the potential to act as a biomarker for the diagnosis of COVID-19 [22].
4.2. Function Enrichment Analysis
In this study, we performed KEGG and GO function enrichment analyses for both upregulated and downregulated DEGs to investigate the biological functions of common DEGs. According to KEGG analysis, the upregulated DEGs were enriched in the B cell receptor signaling pathway, proteoglycans in cancer, and lysosomes, and the downregulated DEGs were enriched in the circadian rhythm, spliceosome, and TGF-beta signaling pathway.
Regarding GO functional enrichment analysis, the BPs of the upregulated DEGs were mainly enriched in response to organic substances, in response to stimuli, and in the inflammatory response. Regarding the downregulated DEGs, the BPs were mainly enriched in the cellular response to chemical stimulus, negative regulation of cellular processes, and regulation of multicellular organismal processes. The CCs of the upregulated DEGs were enriched in vesicles, extracellular regions, and extracellular organelles. Regarding the downregulated DEGs, the CCs were enriched in the nucleoplasm, nuclear speck, and nuclear body. MF analysis suggested that the upregulated DEGs were predominantly enriched in protein binding, identical protein binding, and protein-containing complex binding. Regarding the downregulated DEGs, the MFs mainly included blue light photoreceptor activity, organic cyclic compound binding, and activin binding. Investigating the crucial functions and pathways of COVID-19 and OA contributed to understanding the molecular mechanism underlying these two diseases.
4.3. TF and miRNA Analysis
Furthermore, we performed TF and miRNA analyses to examine the associations between DEGs, miRNAs, and TFs. MiRNAs, a class of non-coding RNAs, can destabilize target RNAs to inhibit specific gene expression and closely interact with genes in the progress of osteoarthritis [23, 24]. TFs can bind to the specific sequence of the target gene to activate its expression in diverse biological activities, such as in the metabolism and immune response [25]. An miRNA–target network was constructed to explore the upstream miRNA of hub genes through the miRWalk 3.0 website. We found three miRNAs targeting CDK1, namely, has-miR-6838-5p, has-miR-497-5p, and has-miR-31-5p. It is reported that has-miR-6838-5p facilitates cell growth and is closely correlated with COVID-19 in men [26]. The plasma level of has-miR-497-5p in the acute phase of COVID-19 was positively correlated with the RBD-IgG antibody response in the recovery phase of the disease [27]. Has-miR-31-5p expression is highly elevated in COVID-19 patients and correlates with inflammation disorders [28, 29][REMOVED HYPERLINK FIELD]. Has-miR-497-5p can protect chondrocytes from cell apoptosis caused by IL-1β and can suppress autophagy in OA [30]. Patients with OA show reduced has-miR-6838-5p expression in cartilage-derived mesenchymal stem cells (CMSCs) [31]. However, the relationship between has-miR-6838-5p and OA is still unclear.
Through the TF–target network, six TFs can activate CDK1, including PTTG1, TFDP1, and E2F1. TOP2A is activated only by E2F1. Activation of E2F1 and PTTG1 alleviates cartilage damage and reduces IL-1β-induced inflammation in OA [32]. TFDP1, the main heterodimeric partner of E2F1, is downregulated in the early stage of OA but upregulated in the advanced stage [33].
4.4. Drug–gene and Disease–gene Interaction Analysis
Moreover, we performed drug-gene and disease–gene interaction analyses to predict the drugs and diseases associated with CDK1 and TOP2A. The interaction network demonstrated that nine cancers are closely correlated with the two hub genes, namely, malignant neoplasm of the breast, breast carcinoma, liver carcinoma, malignant neoplasm of the prostate, glioma, leukemia, adenocarcinoma of the lung (disorder), adrenocortical carcinoma, and diffuse large B-cell lymphoma. A recent study confirmed that COVID-19-infected patients with cancer experience more severe symptoms and higher mortality [34]. However, to the best of our knowledge, few studies have addressed the correlations between OA and these nine cancers.
Our work also revealed 28 drugs that could interact with the two hub genes, such as digitoxin, doxorubicin hydrochloride, etoposide phosphate, vincristine sertraline, rucaparib, and fenofibrate. Among them, fenofibrate is the only agent that has been proven to exert its protective effect on OA and COVID-19. Fenofibrate (FN) can relieve cartilage degradation in OA chondrocytes by reducing inflammation and promoting the apoptosis of senescent cells [35]. In the therapy of COVID-19, it is an anti-inflammation drug that inhibits the inflammatory signaling pathway, which effectively alleviates the symptoms of COVID-19 [36]. Moreover, FN reduces both senescence and inflammation and increases autophagy in both aging humans and OA chondrocytes [35].
Through immune cell infiltration analysis, we found that the amount of immune infiltration of the resting mast cells was significantly different compared to the control and disease groups. Meanwhile, the resting mast cells showed a positive correlation with the hub genes of COVID-19 and OA. These immune cells may be associated with the initiation and progression of OA. However, the role of resting mast cells in COVID-19 is not clear either.
4.5. Advantages and Limitations
Altogether, our study possessed several advantages. First, we utilized the samples of COVID-19 and OA from the GEO database to explore the hub genes significantly correlated with these two diseases. Second, we shed light on the pathologic associations between COVID-19 and OA, which might improve the understanding of the molecular mechanism underlying COVID-19 and OA. Third, we screened out nine hub gene-related drugs, which may be available for the treatment of both diseases.
Despite these, there are several limitations to acknowledge. First, we conducted this study based only on the GEO database. The generalizability of the results requires further verification, especially artificial intelligence-based prediction. Second, basic experiments are needed to verify the functions of hub genes and the efficacy and safety of hub gene-related drugs. Third, more effort and further investigation into the molecular mechanisms of OA and COVID-19 are required.
CONCLUSION
CDK1 and TOP2A were successfully identified as hub genes that can serve as markers of novel targeted therapy for patients with OA after COVID-19 infection. Additionally, 1 immune cell (resting mast cells), 9 tumoric diseases, and 28 potential drugs were identified for the potential therapeutic treatment of OA after COVID-19 infection.
KEY POINTS
- Potential molecular mechanisms underlying OA and COVID-19, as well as their interactions based on the gene expression profiles from the GSE171110 and GSE55235 datasets, were first established.
- Two DEGs, CDK1 and TOP2A, were identified as common hub genes of OA and COVID-19 and could serve as novel therapeutic targets for OA patients with COVID-19.
- The interactive associations of common hub genes with resting mast cells, nine tumoric diseases, and 28 potential drugs could provide new ideas for developing practical therapeutic strategies for OA after COVID-19 infection.
DECLARATION
The English language of the article was improved with ChatGPT.
The author generated this text in part with GPT-3, OpenAI’s large-scale language-generation model. Upon generating draft language, the author reviewed, edited, and revised the language to their own liking and takes ultimate responsibility for the content of this publication.
AUTHORS’ CONTRIBUTIONS
It is hereby acknowledged that all authors have accepted responsibility for the manuscript's content and consented to its submission. They have meticulously reviewed all results and unanimously approved the final version of the manuscript.
LIST OF ABBREVIATIONS
Osteoarthritis | = OA |
Coronavirus disease 19 | = COVID-19 |
Differentially expressed gene | = DEG |
Transcriptional factors | = TFs |
Gene Expression Omnibus | = GEO |
Gene Ontology | = GO |
Protein-protein interaction | = PPI |
Gene set enrichment analysis | = GSEA |
Cyclin-dependent kinase 1 | = CDK1 |
DNA Topoisomerase II Alpha | = TOP2A |
AVAILABILITY OF DATA AND MATERIALS
The data and supportive information are available within the article.
FUNDING
This study was financially supported by the Innovation Capability Enhancement Project of Army Medical University (2022XJS22), the Key Project in Technological Innovation and Development of Chongqing (2022TIAD-KPX0221), the National and Chongqing Continuing Medical Education programs, and a project of Xinqiao Hospital (2022YQB033).
ACKNOWLEDGEMENTS
Declared none.