All published articles of this journal are available on ScienceDirect.
In Vitro and In Silico Study of Nardostachys Jatamansi DC for Cognitive Impairment Related Conditions
Abstract
Aim
This study aims to scientifically evaluate the cognitive-enhancing and neuroprotective properties of Nardostachys jatamansi.
Background
Nardostachys jatamansi (NJ) From the Valerianaceae family, is valued in Ayurveda, and is frequently used as a remedy for a variety of illnesses. These are used in various traditional herbal formulations and nutraceuticals, as well as to treat neurological disorders related to cognitive impairment (CI).
Objective
The purpose of the study was to produce high-quality scientific data regarding cognitive-enhancing and neuroprotective behavior, using in-silico and in-vitro methods. Volatiles including valerenic acid were used for in-silico studies, whereas jatamansi extract was evaluated using High-Performance Thin-Layer Chromatography (HPTLC).
Methods
High-performance thin-layer Chromatography (HPTLC) was used to quantify valerenic acid in NJ extract. Bioinformatics tools were employed to predict the targets and druggability of NJ volatiles associated with neurogenesis and neuroprotection.
Results
The valerenic acid content in NJ02 was found to be 2.65% using HPTLC. In-silico studies revealed the affinity and stable complex formation of valerenic acid, Isoeugenol, and Eugenol with their respective targets GBA1, DRD3, and ERAP1, which are known to play a role in neuroprotection.
Conclusion
The findings provide scientific evidence for NJ neuroprotective and cognitive-enhancing capabilities, supporting its traditional use. This study not only highlights the plant’s potential benefits but also identifies specific phytochemicals that contribute to these effects.Our research emphasizes the need for further studies to explore these phytochemicals in detail and establish optimal non-toxic dosages for clinical applications related to cognitive impairment. These contributions are crucial for advancing the understanding and therapeutic application of NJ in the context of cognitive health.
1. INTRODUCTION
In the global landscape, dementia-related disorders are causing growing concern. In 2015, around 46 million people were affected, but projections suggest this number could rise to 131.5 million by 2050 [1], with Alzheimer's disease accounting for 80% of cases. The disease's causes include abnormal amyloid plaque accumulation, tau protein aggregation, oxidative stress, metal ions, and acetylcholine decline [2]. The disease's complexity highlights its multifaceted nature. As a result, the research is focused on developing effective preventive and treatments, with a significant body of research underway in medication development.
Numerous clinical studies are presently in progress, investigating a range of compounds designed to address different facets of Alzheimer's disease pathology. These studies encompass substances targeting the aggregation of amyloid beta (Aβ), β-secretases involved in the processing of amyloid precursor protein (APP), and agents that modulate tau phosphorylation [3]. The diversity of these approaches underscores the intricacy of Alzheimer's disease and the necessity of multifaceted research efforts. While exploring potential treatments, it's important to note that cholinesterase inhibitors (ChEIs) marked a significant milestone in Alzheimer's pharmacological therapy as they were the first medications to receive approval from the US Food and Drug Administration (USFDA). These ChEIs, including tacrine, galantamine, rivastigmine, and donepezil, have undergone extensive placebo-controlled studies. These investigations have shown that people with mild to severe Alzheimer's disease had somewhat improved symptoms [4]. However, it's crucial to acknowledge the limitations of these medications. Most of them operate through a single target approach, which means that when patients discontinue their use, their Alzheimer's disease symptoms tend to return. Additionally, these drugs have been associated with adverse side effects, underscoring the need for further research into more effective and safer treatment options for this challenging neurodegenerative condition. The ongoing clinical studies mentioned earlier are part of these efforts to find more comprehensive and enduring solutions for Alzheimer's disease.
Ayurveda, a holistic approach to healthcare, uses phytochemical-rich herbs to prevent and manage conditions like dementia. Its historical roots and compatibility with traditional and allopathic systems make it a valuable asset in cognitive health [5]. Ayurveda's “rasayana” herbal preparations, rich in phytochemicals like alkaloids, saponins, flavonoids, terpenoids, and polyphenols, are particularly promising for cognitive health. These compounds reduce oxidative stress, combat inflammation, exhibit neuroprotective effects, and improve blood flow to the brain. Notable herbs like Brahmi, Ashwagandha, and Jatamansi are highly regarded for their cognitive function enhancement [6].
Among these, NJ., a medicinal plant belonging to the Valerianaceae family, is particularly noteworthy. It is native to the Himalayan region and has a wide array of pharmacological properties. In Ayurveda, the roots and rhizomes of NJ have been incorporated into various herbal formulations and nutritional supplements. Jatamansi is renowned for its beneficial effects on cognition and its ability to protect the nervous system. The primary components of this herb are its roots and rhizomes [6]. In Ayurvedic practice, the rhizomes of jatamansi are predominantly utilized as an anticonvulsant, for the treatment of conditions such as hysteria, syncope epilepsy, and mental weakness, and as an anti-stress remedy [7]. The primary active ingredients are volatile, separated, and purified from NJ. These molecules have been extensively researched for their biological properties in a variety of pharmacological domains, including immunomodulatory, anti-inflammatory, analgesic, and anti-tumor actions [8]. The integration of in-silico methods with traditional laboratory techniques is a powerful approach to the study of phytochemicals and their potential impact on biological pathways.
The current study aims to identify the potentially active components against CI in the volatile substances of NJ and to predict their underlying mechanisms of action using a network pharmacology approach. NJ, constitutes the main active molecule of valerenic acid Which will be validated by High-Performance Thin-Layer Chromatography (HPTLC).The study will help us predict the mode of action and target proteins for the potential druggable NJ volatile molecule, which can be further explored and used for drug development against CI-related disorders. Our study lays the groundwork for future studies and clinical trials, aiming to develop new treatments for CI-related conditions.
2. MATERIALS AND METHODOLOGY
2.1. In-silico Studies
2.1.1. Comprehensive Information on Various Phytochemicals in NJ
An in-depth search was done to phytochemical evaluation in NJ utilizing a variety of databases, including PubMed, Google Scholar, Research Gate, Science Direct, and other resources. Research publications published between 1960 and 2024 were the source of the data for this study. ‘ Nardostachys jatamansi phytochemicals’, ‘jatamansi phytochemical’, and ‘ Nardostachys jatamansi Phytochemistry’ was used as the keywords for the search. A list of phytochemicals was prepared from the obtained literature. Another list of volatile compounds was prepared from relevant databases [9]. The two lists were compared to obtain the list of volatile of NJ for the study. CID/SMILES and 3D Structures for the listed compounds were programmatically obtained from the PubChem Database. The method Outline of in-silico study is demonstrated in Fig. (1)
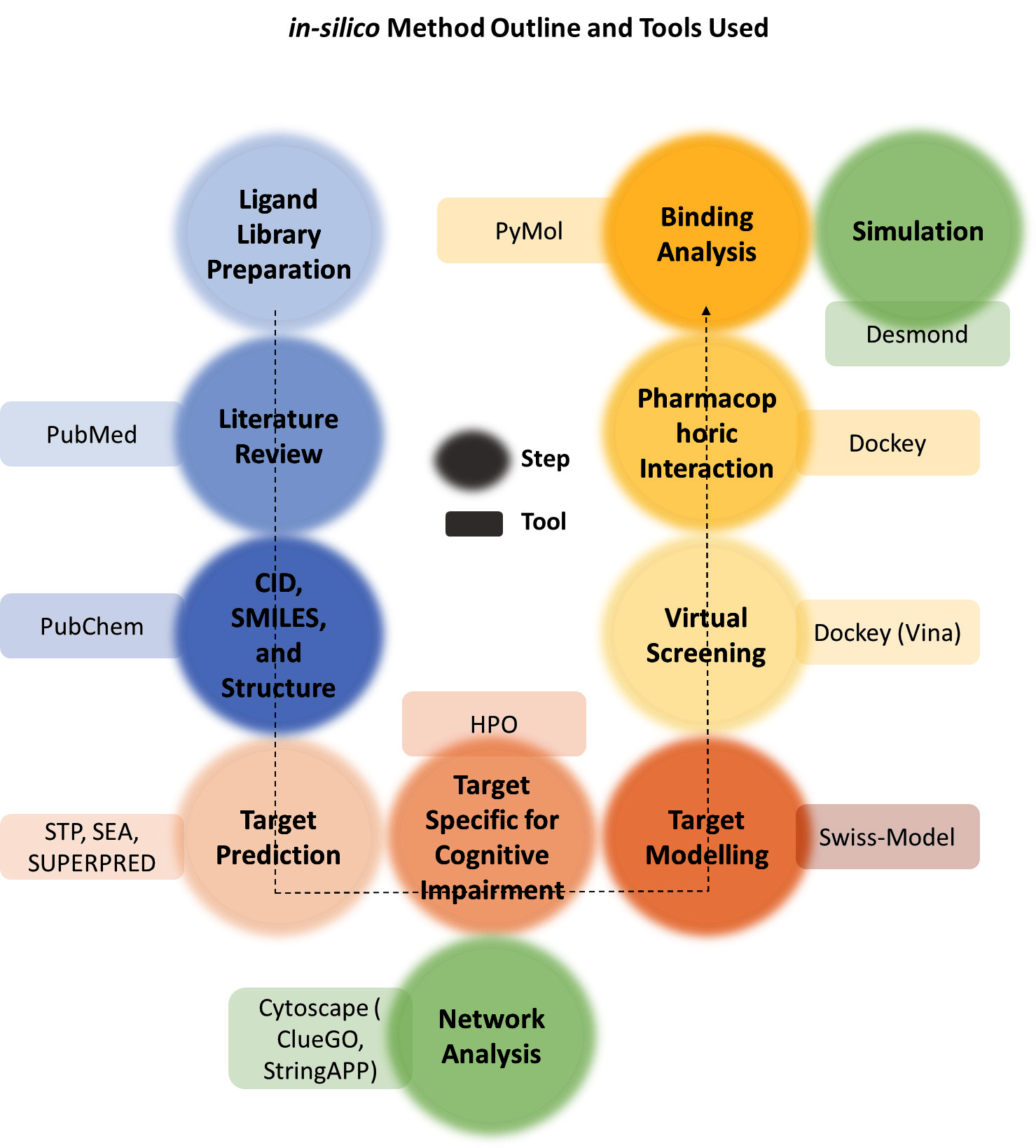
2.1.2. Target Prediction
SMILES obtained from PubChem were used for the prediction of Targets from three different servers, i.e., Swiss Target Prediction [10], Superpred [11] and SEA [12]. Predicted targets were sorted and filtered based on Prediction Score and Model accuracy. The list of targets was compared with the list of genes involved in the CI, obtained from the HPO database. Common genes were used for further study.
2.1.3. Homology Modeling
The gene products i.e., proteins were modeled using SWISSMODEL, where the amino acid sequence of Target proteins was obtained from Uniprot database, and the suggested templets with more than 90% sequence similarity and 75% coverage were used for modeling the Structure [13]. The structures with more than 75% completeness [14] were prepared for Docking Studies.
2.1.4. Network Analysis and Cross Talk of the Targets
Pathway analysis and cross-talk assessment of the identified targets were conducted. This involves the examination of interconnected signaling pathways and interactions among the molecular targets. The objective was to gain a comprehensive understanding of how the identified compounds could potentially modulate various pathways, thus contributing to their multifaceted effects on cognitive function and central nervous system disorders. This study was performed using Cystoscope [15] with ClueGO [16], StringApp apps [17].
2.1.5. Docking Studies
The ligands were prepared for Docking studies, in the Dockey platform. All the Ligands from the prepared library were docked on each of the optimized protein structures using Autodock Vina in Dockey [18].
2.1.6. Molecular Dynamics
The Desmond Schrodinger 2023.2 suit was used to do the docking calculation [19] with the optimal binding affinity compound. In this research, molecular dynamics simulations were employed to study a complex involving a specific compound and a protein. The simulations were run using membrane proteins to simulate physiological circumstances at a constant temperature of 300 K and pressure of 1 bar. Molecular interactions were simulated using the OPLS_2005 force field, and electrostatic charges were computed with the Ewald technique. To get extremely fine data, itineraries were taken at 4.0 picosecond intervals. The study aimed to analyze the behavior of both the compound and the protein. A specialized analysis tool within the Desmond package was used to investigate their interactions. Complex stability was assessed through two key metrics: Root Mean Square Deviation (RMSD), it measured how the complex's structure deviated from a reference state, and the interaction between the ligand and the protein over 100ns.
2.2. In Vitro Analysis
2.2.1. Plant Collection
Three different samples of NJ dried rhizomes were procured and validated from the Heartyculture Nursery, Kanha Shanti Vanam, Telangana. The three samples were named MGMNJ01, MGMNJ02, and MGMNJ03 The analytical grade chemicals and reagents used were all acquired from TCI Chemicals, Tokyo.
2.2.2. Developing the Ethanol-based NJ Extract
NJ rhizomes were cleaned, dust particles removed, dried, ground, and extracted using a Soxhlet method as per Indian pharmacopia. The solvent was evaporated, and stored at -20°C, and the dried extract was dissolved in distilled water.
2.2.3. Making the Standard Solution
A functional solution of 100 mg/mL was produced by combining 900 mL of analytical-grade methanol with 100 mL of the stock liquid of valerenic acid. The base solution of valerenic acid (1 mg/mL) was created by dissolving 1 mL of tightly packed valerenic acid in 1 mL of methanol.
2.2.4. Chemo-profiling using High-performance Thin-layer Chromatography
A 10 μL specimen solution was placed on a silica gel-coated plate, air-dried, and placed in a glass chamber with a mobile phase. Over 30 minutes, the mobile phase separated components based on polarity and affinity. A CAMAG TLC scanner was used to scan the plate at 620 nm, creating chromatograms plotting peak intensity against the retention factor (Rf). The CAMAG visualizer was used to observe and document band characteristics. Win CATS software generated peak tables for component identification and quantification [20]. An initial phytochemical evaluation was performed to check for additional metabolites.
3. RESULTS
3.1. In-silico Studies
3.1.1. Ligand and Targets
This study used databases like Swiss Target, SEA, and Supered Prediction to identify 628 target genes linked to 14 active ingredients. A score requirement of at least 0.6 was used to ensure high-confidence projected targets. A total of 644 genes associated with CI were retrieved from HPO databases. Venn diagram evaluation identified common targets shared by drugs and CI genes. 15 putative CI-associated genes unique to NJ volatiles were identified (Fig. 2), emphasizing their importance in the context of CI and potential therapy benefits (Table 1).
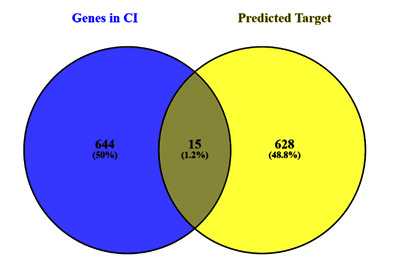
Phytochemical Name | Structure | Number of Targets |
---|---|---|
3-Methylbutanoic acid | ![]() |
98 |
α-Cadinol | ![]() |
68 |
Carotol | ![]() |
79 |
Dodecane | ![]() |
144 |
Eugenol | ![]() |
207 |
Isoeugenol | ![]() |
190 |
L-Calamenene | ![]() |
81 |
Linalool | ![]() |
55 |
Palmitic acid | ![]() |
319 |
Patchouli alcohol | ![]() |
86 |
trans-Nerolidol | ![]() |
66 |
α -ionone | ![]() |
60 |
β-patchoulene | ![]() |
79 |
Valerenic Acid | ![]() |
48 |
Gene | Full Form |
---|---|
AKT1 | Protein kinase B (Akt serine/threonine kinase 1) |
CCR1 | C-C chemokine receptor type 1 |
DRD3 | Dopamine receptor D3 |
EP300 | E1A binding protein p300 (p300) |
ERAP1 | Endoplasmic reticulum aminopeptidase 1 |
FAS | Fas cell surface death receptor (CD95) |
GBA1 | Glucosylceramidase beta 1 (acid beta-glucosidase) |
MTOR | Mammalian target of rapamycin |
NOS3 | Nitric oxide synthase 3 (eNOS) |
PLK4 | Polo-like kinase 4 |
SCN2A | Sodium voltage-gated channel alpha subunit 2 |
SCN3A | Sodium voltage-gated channel alpha subunit 3 |
SGPL1 | Sphingosine-1-phosphate lyase 1 |
TERT | Telomerase reverse transcriptase |
TLR4 | Toll-like receptor 4 |
3.1.2. Homology Modelling
All these 15 targets (Table 2) were modelled, to fill in the gaps and remodel the loops. The 10 structures found to have ≥75% of amino acid residues (i.e., CCR1, DRD3, ERAP1, GBA1, MTOR, NOS3, SCN2A, SCN3A, TERT a TLR4) were taken for Docking and Molecular Dynamics studies and remaining were only used in network analysis.
3.1.3. Network Analysis and Cross Talk of the Targets
3.1.3.1. PPI Network Construction
We used Cystoscape to analyze the interactions between compounds and their targets. And found a complex web of interactions, with different pathways linked to different ligands through intermediary genes. The compound-target network study revealed possible synergistic effects of projected targets, suggesting this plant could help fight disease by providing important information about how volatile phytochemicals interact with their targets. This visualization supports the idea that these plants could help fight disease by providing valuable insights into the interactions between volatile phytochemicals and their targets as depicted in Fig. (3).
3.1.3.2. Enrichment Analysis Results
A study was conducted to investigate the functions of 15 potential CI targets of volatile substances, such as valerianic acid, found in NJ. The 15 target genes showed significant enrichment in biological processes (BP), cell compartments (CC), and molecular function (MF). The top three BP phrases were a reaction to oxygen levels, neuron death, and cell response to oxygenation levels. The top three CC keywords were voltage-gated sodium channel combination, sodium channels complex, and endocytic vesicles. The top three MF phrases were sodium channel activity, calmodulin binding, and voltage-gated sodium channel function. 146 pathways could be impacted by NJ volatiles, with the most prominent being hepatitis B, Kaposi sarcoma-associated herpesvirus infections, and the HIF-1 signaling pathway. Ten outstanding routes and the top ten GO functional groups in BP, CC, and MF were chosen for review (Fig. 4).
3.1.4. Molecular Docking Analysis
Our study also focused on assessing ligand-receptor binding affinity by correlating it with ligand efficiency (Fig. 5a, 5b), LogKi values (the logarithm of the inhibition constant), and the presence of hydrogen bonds as well as interaction sites. We set stringent criteria, selecting complexes with binding affinities, ligand efficiency, and LogKi values exceeding -4 kcal/mol, 40, and -4.5, respectively, for further investigation. Specifically, 7 complexes outlined in red in Fig. (5a) were earmarked for subsequent in-depth studies which are represented in Table 3.
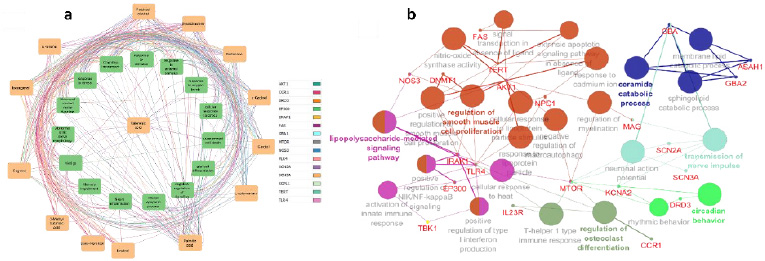
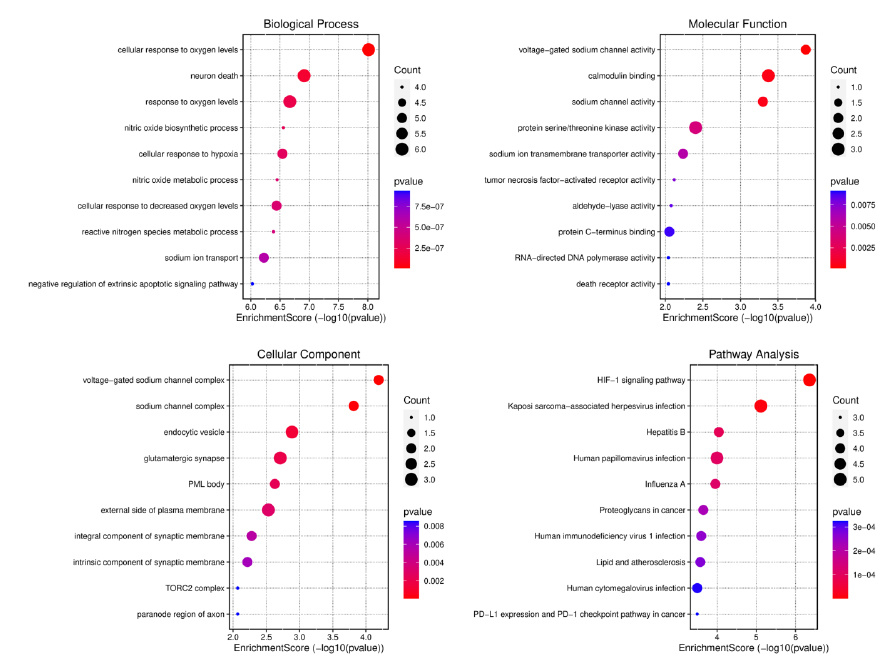
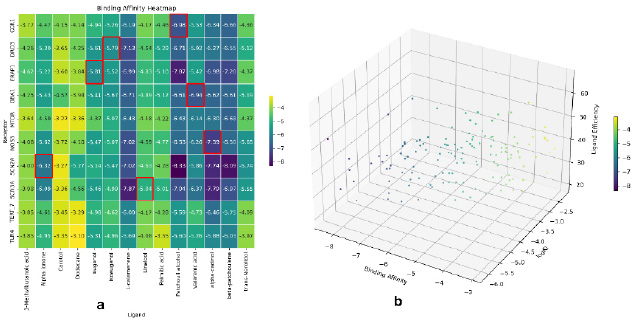
Receptor | Ligand | Affinity (kcal/mol) | FQ | LE | SILE | LELP | Ki |
---|---|---|---|---|---|---|---|
CCR1 | Patchouli alcohol | -6.979 | 0.786 | 0.436 | 3.038 | 8.279 | 7.66 uM |
DRD3 | Isoeugenol | -5.792 | 0.722 | 0.483 | 2.748 | 4.063 | 56.81 uM |
ERAP1 | Eugenol | -5.812 | 0.724 | 0.484 | 2.758 | 4.055 | 54.92 uM |
GBA1 | Valerenic acid | -6.94 | 0.77 | 0.408 | 2.966 | 7.431 | 8.18 uM |
NOS3 | α-Cadinol | -7.388 | 0.833 | 0.462 | 3.216 | 8.346 | 3.84 uM |
SCN2A | α -Ionone | -6.317 | 0.741 | 0.451 | 2.862 | 8.004 | 23.42 uM |
SCN3A | Linalool | -5.043 | 0.657 | 0.458 | 2.456 | 6.493 | 201.11 uM |
Proteins exhibit distinct binding patterns with their respective ligands. CCR1, a receptor protein, forms hydrophobic interactions with Patchouli alcohol at residue sites 113, 114, and 185, involving tyrosine and leucine amino acids. DRD3 forms essential hydrogen bonds with Isoeugenol at residue sites 192 and 349, using serine and histidine amino acids for stabilization. ERAP1 engages in hydrogen bonding with Eugenol at residue sites 551, 648, and 921, involving lysine, serine, and tryptophan amino acids. GBA1 forms hydrogen bonds with Valerenic acid at residue sites 408 351 and 355, involving threonine, tryptophan, and phenylalanine amino acids. NOS3 establishes multiple hydrogen bonds with α-Cadinol at residue sites 940, 955, 939, 957, 1159, and 1162, involving serine, threonine, tyrosine, alanine, and phenylalanine amino acids. SCN2A forms hydrogen bonds with α-Ionone at residue 1460, and SCN3A exhibits hydrogen bonding with Linalool at residue 1414, involving alanine and alanine amino acids.
3.1.5. Molecular dynamic simulation
Molecular dynamics simulations provide important information on the stability of complexes including proteins and ligands. As illustrated in Fig. (6), we can observe distinct behaviors among the best-hit molecules following the initial docking. In the case of the GBA1-Valerenic acid complex (Fig. 6d), the binding complex exhibited the most substantial interaction changes, signifying a torsion shift within a stable complex. Conversely, for the DRD3-Isoeugnol complex (Fig. 6b) and the SCN3A-Linalool complex (Fig. 6g), we observed shifts in ligand location before attaining a stable structure. Additionally, for the CCR1-Patchouli alcohol complex (Fig. 6a), ERAP1-Eugenol complex (Fig. 6c), and NOS3-α-Cadinol complex (Fig. 6e), the initial interactions were to some extent preserved. Notably, the Root Mean Square Deviation (RMSD) in all these complexes reached a plateau after approximately 40 nanoseconds (ns). This observation suggests that all the aforementioned complexes are stable and do not undergo significant conformational changes in the protein structure during the simulation.
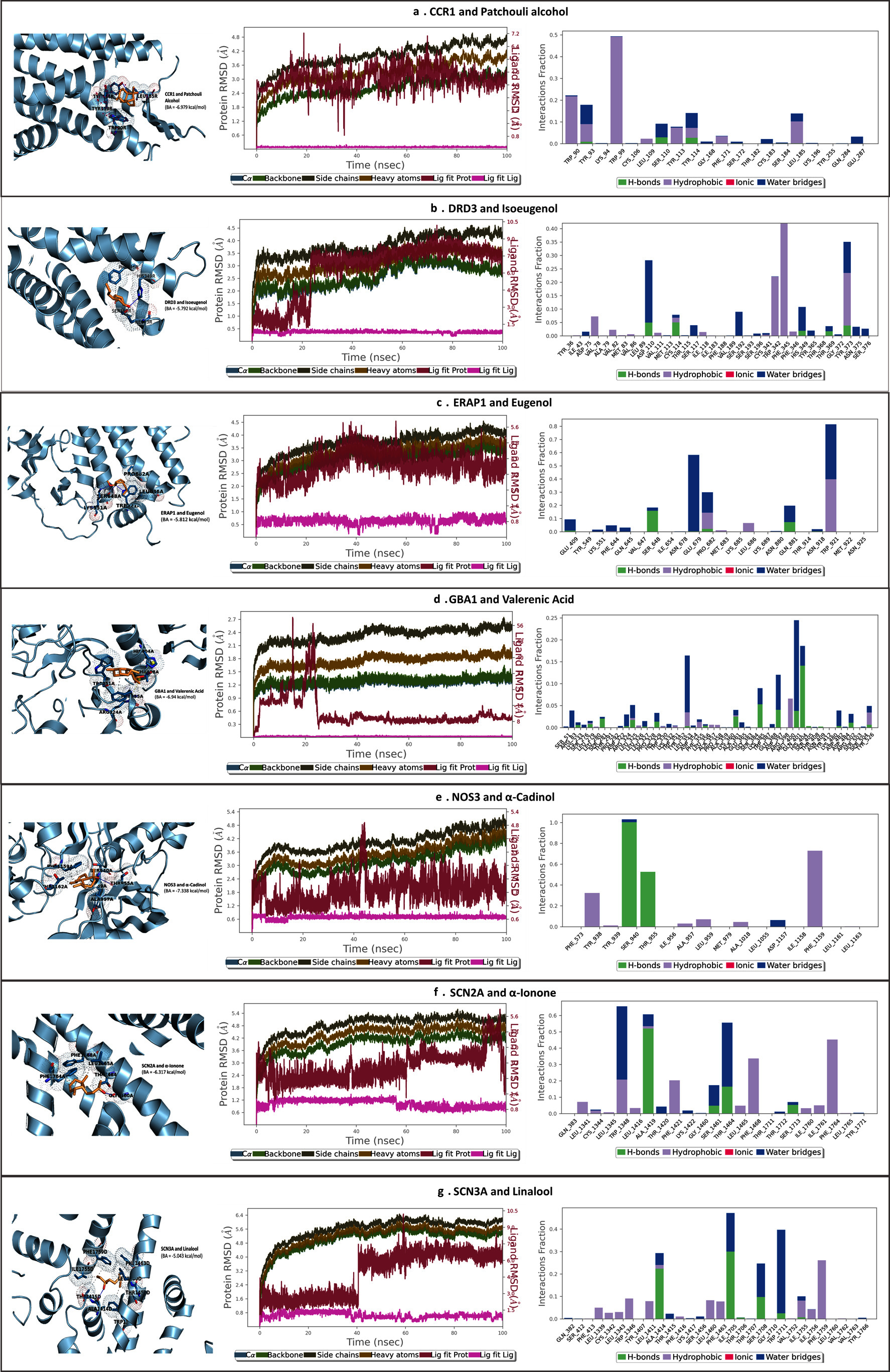
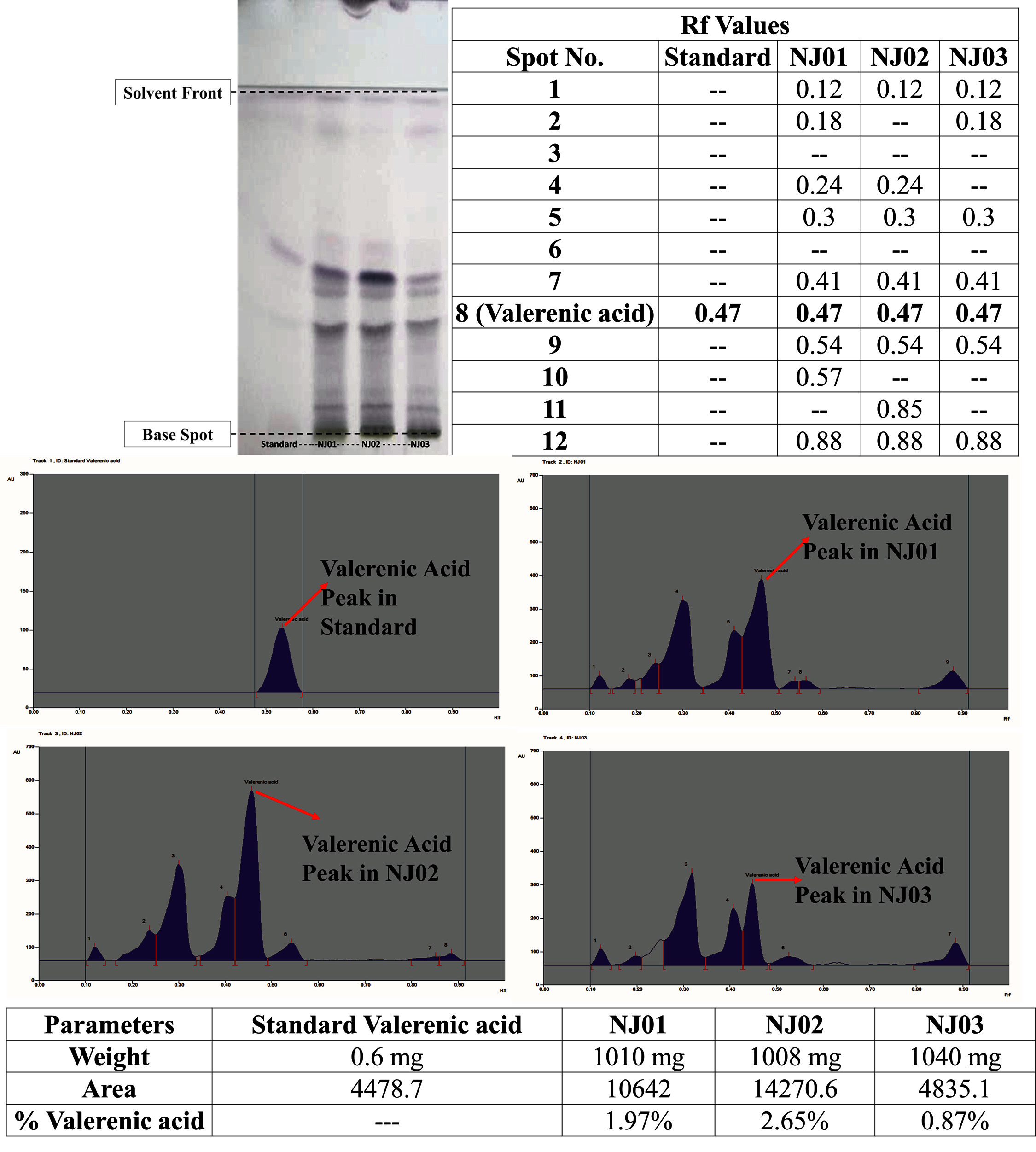
– (a) Fingerprint of Samples against standard Table, (b) Rf values of various components in extracts, (c) Chromatograph of Standard, (d) Chromatograph of NJ01, (e) Chromatograph of NJ02, (f) Chromatograph of NJ03, (g) Calculated percentage composition of valerenic acid in samples
3.2. HPTLC Profile
The study tested methanolic extracts from three samples (MGMNJ01, 02, 03) for phytochemical analysis of NJ (Fig. 7). The mobile phase provided compact bands for phytochemical ranges. The method effectively separated and resolved standard valerenic acid peaks, confirming the quantification of three samples. Densitograms showed characteristic valerenic acid peaks for all samples. The percentage composition of valerenic acid was calculated with weight and area.
4. DISCUSSION
As the population ages, cognitive impairment is becoming a more pressing problem in medical consultations. Dementia, the most severe type of cognitive impairment, is typified by cognitive deficiencies that make it difficult to carry out daily duties and ultimately result in a loss of independence [7]. For millennia, conventional Indian medical systems such as Ayurveda and Unani herbal remedies have been utilized for the prevention and treatment of illness. These systems focus on methodical techniques that address various elements and parts. Herbal medicines have long been utilized as an alternative to conventional treatments for illnesses linked to cognitive decline. Notably, NJ is frequently used as an anticonvulsant and as a stress reliever for ailments like hysteria, syncope epilepsy, mental weakness, and so forth [6].
NJ comprises a diverse array of active phytochemical components that influence various targets and pathways associated with cognitive impairment (CI). In this study, one of these bioactive compounds, specifically valerenic acid from NJ, was chosen for investigation. However, it is still uncertain whether the compounds found in NJ can effectively address CI in patients. To gain insights into the molecular mechanism, this research employed network pharmacology, molecular docking, and simulation techniques. Additionally, in vitro analysis using HPTLC was conducted to validate the presence of valerenic acid in the rhizobium of NJ sourced from three different plants.
The study forecasted 628 shared targets associated with volatile compounds found in NJ and extracted 644 proteins related to cognitive impairment (CI) from the HPO database. Comparative analysis revealed 15 common genes in both sets. When enriched they showed their roles in functions and pathways associated with oxidative stress, nitric oxide metabolism, and neurodegenerative processes. Besides valerianic acid, we identified 8 potential ligands for CI-related target proteins, aiding future research into the mechanism and patient therapy. Valerianic acid targets, such as SCN2A and TLR4, play crucial roles in nerve impulse transmission, cell proliferation, and differentiation, essential for a healthy brain [21-23].
The intricate pathogenesis involves key biological pathways such as inflammation, oxidative stress, apoptosis, autophagy, lipid metabolism, and circadian rhythm. Amyloid beta and tau production and clearance pathways, driven by ubiquitin-proteasome system, signal peptides, and F-box proteins, offer potential targets to mitigate plaque and tangle formation in Alzheimer’s. Pathways tied to blood-brain barrier integrity, neurovascular unit, and synaptic transmission, modulated by NOS, type I interferons, TLR4, CCR1, SCN2A, and SCN3A, could enhance cerebral oxygenation, reduce inflammation and oxidative stress, and bolster memory processes [24]. Hence, precision modulation of these pathways may restore brain molecular balance and homeostasis, ultimately benefiting cognitive function.
Both GO and KEGG enrichment analyses were performed on the 15 potential targets. The outcomes of the GO analysis revealed that there were cell reactions to changes in oxygen consumption, neuronal dying, voltage-gated sodium channel multifaceted, endocytic vesicles, and calmodulin binding. These all are interconnected in the realm of neurobiology and cognitive function. Oxygen is vital for neurons, and its deficiency can lead to neuron death or neurodegeneration [25, 26]. The voltage-gated sodium channels are crucial for neuronal communication, and any dysfunction can affect cognitive processes. Endocytic vesicles are involved in the internalization and recycling of molecules, influencing signal transduction and cognitive function [26]. Calmodulin binding is essential for signal transmission within neurons, affecting processes like memory, learning, and information processing [27]. Therefore, these components are all critical for maintaining healthy neuronal function and cognitive processes.
Molecular docking results confirmed that Patchouli alcohol binds at the active site of CCR1 with a high affinity. CCR1 is known to interact with cytokines and participate in control of neuroinflammation [28]. DRD3 is another protein involved in neuroinflammation [29] which shows a higher affinity for Isoeugenol. ERAP1 with affinity for eugenol, is known to play a role in tumorigenesis [30]. GBA1 responsible for ceramide biosynthesis and plays a crucial role in the myelination of neurons has shown an affinity for Valerenic acid. NOS3 has an affinity for α-Cadinol. Whereas SCN2A and SCN3A, the sodium channels have affinity for α -Ionone and Linalool respectively. The significance of NOS3 and sodium channels is briefly discussed earlier, they play a crucial role in nerve impulse conduction and maintenance of oxidative stress in neurons. The Molecular Dynamic simulation suggests that all the aforementioned complexes are stable and do not undergo significant conformational changes in the protein structure during the simulation of 100ns.
Though, the pharmacological potential of individual phytochemicals as are very promising, it is essential to quantify their presence in the crude drug (extract), for proper dosage estimation and development. Hence, researchers employed HPTLC for the measurement of valerenic acid from the Methanolic extracts of NJ from each sample (MGMNJ01, 02, 03) the findings are provided in Fig. (7b). For valerenic acid, the correlation coefficient and Rf value have been determined to be 0.998 and 0.36, respectively. Above Insilco studies have proven that valerenic acid is also involved in various genes for cognitive impairment. In a comparable direction, a small number of studies have shown that NJ extract (200–600 mg/kg body weight, orally) enhances mouse learning and memory [31] and ameliorates other neurodegenerative cascades in rats [32, 33]. Numerous researchers have used numerous animal models to study this species' capacity to relieve stress [34]. It was shown that the extract could control the activities of catalase, nitrite, superoxide dismutase, and lipid peroxidation. This shows that the extract's ability to reduce stress may be due to its antioxidant qualities. More research showed that valerenic acid, a sesquiterpenoid, is an important sign of the Valerian plant that is connected to the brain and spinal cord [6]. The NJ extracts' neuroprotective efficacy is most likely attributable to their comparatively high valerenic acid concentration. This partially substantiates the traditional usage of NJ for cognitive enhancement [35] and enhanced neurotrophic function [36].
LIMITATIONS
Firstly, being a bioinformatics study, even though we have shortlisted a few phytochemicals as a potential druggable candidate, it must be validated in the wet lab and clinical settings. Secondly, we have selected NJ from only one region; further studies with samples from different geographical and climatic regions can help in the generalizability of our findings.
CONCLUSION
The study analyzed volatile compounds in NJ, a herbal plant known for its many medicinal qualities, which are linked to its bioactive phytochemicals, such as valerenic acid. Using an integrated approach including target prediction and network analysis, multiple protein targets were identified, showing implications on a range of biological processes, including neuromodulatory pathways. The molecular docking and simulation studies provided insights into binding affinities and interactions, revealing their potential biological activities. HPTLC was used to validate the presence and concentration of volatile compounds, enhancing the analytical methods' reliability.
Our study explores the potential therapeutic applications of volatile compounds, laying the groundwork for further experimental validation and drug development. The study also suggests exploring the synergistic effects of volatiles in combination with other bioactive compounds, potentially leading to novel multi-targeted therapies. The insights from this study highlight the potential of using natural plant volatiles as therapeutic agents. Future research could focus on detailed pharmacokinetics and pharmacodynamics, assessing their efficacy and safety in clinical settings. The integration of computational and experimental approaches serves as a blueprint for designing plant-based therapeutics. The findings emphasize the importance of interdisciplinary research in unlocking the therapeutic potential of natural products, ultimately improving health outcomes and quality of life.
improving health outcomes and quality of life.
AUTHORS CONTRIBUTION
The authors confirm their contribution to the paper as follows: study conception and design: K.S.T., Y.P.; analysis and interpretation of results: S.A.; draft manuscript: A.K., S.S.; Experiment Conducted: P.P.; Principal Investigator and Study Supervisor: M.T. All authors reviewed the results and approved the final version of the manuscript.
LIST OF ABBREVIATIONS
USFDA | = US Food and Drug Administration |
ChEIs | = cholinesterase inhibitors |
APP | = amyloid precursor protein |
HPTLC | = High-Performance Thin-Layer Chromatography |
CI | = cognitive impairment |
NJ | = Nardostachys jatamansi |
AVAILABILITY OF DATA AND MATERIALS
The data and supportive information are available within the article.
ACKNOWLEDGMENTS
Special thanks to CRL Lab, MGMSBS, and MGMIHS, for providing the infrastructure. The authors would like to thank Vasu Research Centre, Vadodara, Gujarat for providing an HPTLC facility for related studies.